It is typical for decisions on the management of surface water quality to impact several environmental,
social, and economic factors or
attributes important to the public.
In theory, this can lead to a
difficult and complex problem analysis, but in practice many factors are virtually ignored during analysis and decision making. Further, it is typical for the
predictions of the impact of proposed management actions on these attributes to be highly uncertain.
Often it is possible to reduce that uncertainty through additional research and
analysis, but resources for this are scarce and decision makers may
not be inclined to wait for these results as
they seek action and quick problem solution.
In part to provide the scientific
basis for problem solution and
action, a variety of simulation models have been developed, yet
increasingly it is being recognized that these models are not very
reliable. As a consequence, whether it is the initial intent or not, successful
management often involves judgment-based decision, followed by implementation, feedback, and readjustment.
This “learning by doing” approach is a pragmatic
attempt to deal with growth, change, new information, and imprecise forecasting.
This adaptive approach may be particularly appealing in
situations where population growth,
land use change, and variability in climatic
forcing functions exceed the limited
realm of current observation and experience. Such systems involve complex and often highly nonlinear relationships among
various elements; prediction in
these chaotic environments can be
difficult in the short term and useless in the long term. Even state-of-the art models of such systems require
periodic observation, evaluation, and revision in order to improve predictive validity.
Consider the USEPA TMDL program, where the often-substantial
uncertainties in water quality forecasting, the practical difficulties in comprehensive error estimation and propagation, and the
inadequate approaches
for TMDL margin of safety estimation are each compelling reasons to consider a new approach to TMDL assessment and implementation. These problems
are not merely of academic
interest; rather, they are indicative of flawed TMDL forecasts, leading to
flawed TMDL plans. The consequence is
that TMDLs will often require re-adjustment.
These forecasting difficulties formed the basis for the 2001 NRC panel recommendation for adaptive implementation
of TMDLs. Stated succinctly, if there is a good chance that the initial forecast will be wrong, then an appropriate
strategy may be one that, right from the start, takes into account the
forecast error and the need for post-implementation
adjustment. Decision analysis provides a prescriptive framework for this type of assessment.
In a decision analytic framework, scientific research and monitoring studies in support of environmental
management should be selected on the
basis of an assessment of the value
of information
expected from completion of the study.
In general, scientific investigations should be supported if the value of the expected reduction in prediction uncertainty appears to justify the cost of the
scientific analysis. In a formal decision analysis, one could estimate
the change in expected utility associated with the cost of additional investigations, the deferral of decision making until new analyses are undertaken, and the reduction in prediction uncertainty.
An assessment
of cost versus uncertainty reduction can be made
in a formal, rigorous sense using decision analysis. If a simulation model is selected as the predictive framework for TMDL development, sensitivity analysis might be applied with the model to estimate
the relative magnitude of uncertainties. Alternatively, if sensitivity
analysis is not feasible, expert judgment may
be used in conjunction with the model
to define scientific uncertainties that appear to have the greatest impact
on prediction error. If cost is acceptable, research and/or monitoring to improve scientific
understanding may then be directed
toward reducing those uncertainties and ultimately updating the model forecast.
An expected utility framework is proposed to relate prediction
uncertainty reduction (weighted by
the probability of successful completion
of the project), project cost, and other research
evaluation criteria on a common
valuation scale. The result is a priority list for proposed scientific research and monitoring activities that can support an
analytic approach for adaptive implementation of a TMDL. While this example is presented within the framework of an adaptive
TMDL, it is important to emphasize that it is quite general;
indeed, the example below was actually conceived and proposed for
the South Florida Water Management
District outside of the TMDL program.
Water
quality standards, which consist of a designated use and a criterion, provide
the foundation for a TMDL. If measurement of the criterion indicates that a standard is violated, then a TMDL must be developed for the pollutant causing the violation.
In most cases, a water quality model is used to estimate the allowable pollutant loading to achieve compliance with the standard.
The criterion of interest here, chlorophyll a concentration, may be affected by a number
of management options; certainly one
of the more likely options for limitation of algal concentration is to regulate
land use for nutrient load controls. Now, in order to predict the effect of a land use control option on
chlorophyll a, the scientist must link land use and chlorophyll
in some way (perhaps through a mechanistic model, or through a statistical model, or through
expert judgment). The analysis
presented here is based on the assumption
that this link for Lake Okeechobee
eutrophication management will be
the simulation model WASP.
Thus, WASP provides the comprehensive
predictive framework (when linked to
an assessment
of the relation between land use and nutrient loading to the lake) for
assessing the impact of management
actions on the chlorophyll criterion. Any proposed scientific investigation (research and monitoring) designed to improve
the prediction of the impact of management decisions should be selected using WASP as the evaluation framework.
Also, research should not be guided
toward scientifically interesting questions or motivated by the desire simply
to improve
scientific understanding, unless this additional scientific knowledge is
justified in an evaluation using the
WASP predictive framework.
For example, ecologists may
be quite uncertain concerning the role of zooplankton grazing on phytoplankton density and chlorophyll a. Further, it may be believed that this phytoplankton loss term is extremely
important in some lakes. Formal or
informal analysis of research projects using the predictive framework (e.g., WASP) may then be
used to determine:
- Can research successfully be completed within a specified time frame to reduce uncertainty concerning the effect of zooplankton grazing on chlorophyll in the lake?
- What is the expected reduction in prediction uncertainty, for the chlorophyll criterion, associated with a proposed zooplankton grazing research project?
- Is the uncertainty reduction versus cost ratio for this project favorable relative to other proposed research and monitoring projects?
The utility-based method proposed below may be used to conduct this analysis.
In summary, for this case study on Lake Okeechobee, an expected
utility framework is proposed to assess the value of monitoring/research in improving predictions. Application of this approach is quite general; it could serve
to prioritize studies for initial model development,
or it could be used for iterative improvement of a model and its forecast, as in an adaptive TMDL.
If it is assumed that uncertainty reduction and project cost are judgmentally independent, then an additive utility model (see the equation below) may be a reasonable approximation for the valuation model. Judgmental
independence may be assumed for two attributes if value judgments
can be made about each attribute
separately. For example, project cost
and uncertainty reduction are
usually related, since project options can often be identified for which uncertainty reduction increases with
project cost. However, it is likely that judgments
(not tradeoffs) concerning the
relative value (or loss) due to project cost, and the relative value of
uncertainty reduction, can be made
separately. Thus, for a particular scientific research or monitoring
project, aj, the expected utility (EU)
of the project is estimated as:
where Ui(xi) is a utility measure (i.e., a scale reflecting value) for the ith attribute, which
might be uncertainty or cost; wi is a weight on the ith attribute; and pi is the estimated probability that the proposed project will yield the expected level (x) for the ith attribute (e.g., what is the probability that the expected 20% uncertainty reduction will be achieved?). Note that the cost attribute may often have a probability of one (indicating that the estimated project cost is expected to be realized). The additive model in Equation 1 is a reasonable, but certainly not the only, approach to combine information and rank proposals.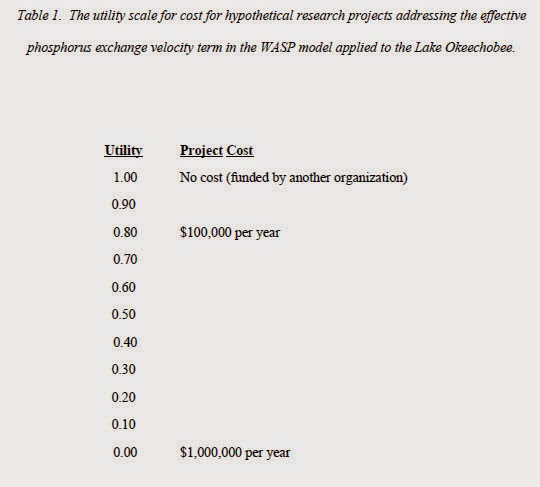
Weights are then assigned to each attribute reflecting relative importance and also reflecting the approximate range on the magnitude of each attribute. Relative importance is simply a measure of the significance of each attribute to the decision. Range is important because, if all projects have exactly the same cost (zero range), then cost is irrelevant as a decision making attribute. Once this general scheme is established, any particular research or monitoring project can be assessed in a straightforward manner.

To begin the project assessment, the level (or magnitude) of each attribute must be
estimated for the project of
interest. For example, research/monitoring cost would be estimated
in the usual way and then converted to the utility scale for cost.
Prediction uncertainty, however, is likely to be more complicated.
First, the uncertainty in the quantity of direct
interest in the proposed research or
monitoring project must be estimated.
Thus, if research is designed to reduce
the uncertainty in the WASP
phytoplankton settling velocity parameter,
the expected reduction in uncertainty in this parameter must be estimated.
Once the parameter uncertainty is estimated,
it must be converted into a
reduction in prediction uncertainty for the attribute (e.g., chlorophyll) of interest. This conversion
may be accomplished with sensitivity analysis using WASP
or may be based on expert judgment by a scientist familiar with WASP and ecological processes
in Lake Okeechobee. Next, the prediction error reduction estimate is converted to the utility scale. The utilities for all attributes are weighted, then multiplied by an estimated probability
of achieving the expected attribute
level. The final step is summation of the probability-weighted
utilities.
While
these uncertainty estimates and
project success probabilities are useful
measures of the value of research/monitoring,
they are not routinely estimated in research/monitoring design. Nonetheless, these terms must
be estimated to carry out the
proposed project evaluation scheme. Scientists may be uncomfortable
with this quantification requirement;
however, they must recognize that their recommendation in support of a research or
monitoring study to guide environmental
decision making is tantamount to a favorable project rating
using a scheme like that in Equation 1. Reduction in
scientific uncertainty and probability of project success are implicit in any project recommendation.
Assume
that the predicted in-lake sediment
phosphorus flux rate based on the application
of WASP to Lake
Okeechobee is quite uncertain, and phosphorus release from the lake
sediments is thought to be an
important contribution to
chlorophyll development. The
expression in WASP for sediment
phosphorus flux rate is (in g/m2-day):
where EDIF is the diffusive exchange coefficient (in m2/day), Dj is the benthic layer depth (in meters), DIP is dissolved
inorganic phosphorus (in g/m3), DOP is
dissolved organic phosphorus (in g/m3), j
refers to the benthic layer, and i refers to the water column. The two uncertain parameters
of interest, EDIF and Dj, are likely to be correlated, and it is likely that their combined
effect can be more precisely estimated than can the combination of their separate effects.
Thus, proposed research is designed
to estimate the quotient of the parameters:
where vp is
an effective phosphorus exchange velocity term
(in m/day).
Based on current knowledge, the uncertainty in vp can be expressed in percent
error, with one standard error estimated
to be about ±40% (i.e., there is about a 2/3 chance that ±40% bounds the error). This error estimate reflects both scientific
uncertainty and natural variability. Research
is proposed involving in situ sediment phosphorus flux studies at randomly selected locations over a three-year period (in the hope of observation during a range of meteorological and hydrological conditions) at a cost of $150,000/year.
Scientists expect these studies to reduce the
error in vp by
one-half (from ±40% to ±20%).
However, the scientists acknowledge that they have a few concerns about
research methods and possible problems in the field, so they have set the probability of research success
(defined as achieving the expected
reduction in uncertainty) at 0.8. The probability that the project will cost
$150,000 as expected is set at 1.0.
While
research is focused on improved
estimation of vp, this research has management interest because it is expected to reduce WASP prediction error for the chlorophyll
attribute. To estimate the prediction error reduction, Monte Carlo simulation can be used with WASP to
determine the effect of a
reduction in error in vp from ±40% to ±20%. Alternatively, expert judgment from a scientist familiar with WASP
and with ecological processes can be substituted if WASP is not
satisfactorily calibrated for the application. The latter option was chosen
here, and the expert estimated
that the expected reduction in vp error would reduce the WASP prediction
error for the chlorophyll attribute by 5%-10%.
All necessary information has been obtained, so
utilities can be estimated and Equation 1 can be applied. Based on
Tables 1 and 2 (which are to represent the value judgments of decision makers), the utility for cost appears to
be approximately 0.7, and the
utility for prediction uncertainty reduction appears to be
about 0.4. The decision maker in
this case assesses the weight on the
prediction uncertainty attribute to be about three times larger than the weight on
cost; therefore, the uncertainty weight is 0.75 and the cost weight is
0.25. Applying the equation, the expected
utility of the project (a1) is:
The utility for all other proposed
projects addressing the chlorophyll attribute can be determined in the same way and then compared to yield a relative ranking on the expected utility
scale. Research and monitoring projects with the highest expected utilities should
have the highest priority for
support.
The analysis that is presented here is hypothetical while still intended to describe how improvements to models might be
rigorously assessed. Nonetheless, one must
acknowledge that it is quite possible that any
individual experiment or monitoring project will contribute only a relatively small amount to
uncertainty reduction. Further, the value of information
calculations described above may be quite uncertain in practice. For
large process models the sensitivity analyses may be difficult, as correlations between model terms
may be important yet difficult to estimate. Nonetheless, as already noted,
the decision to conduct additional monitoring
or research to improve the scientific basis for decisions
carries with it, at a minimum, an implied
acknowledgment that the
benefits of new scientific information justify the costs.
In practice, a number of the choices and issues that make up this analysis are far from straightforward. For example, a proposed project can serve to improve the assessment of compliance with a water quality standard
(i.e., learning) and/or can serve to actually achieve compliance (i.e.,
doing). Thus, some projects (e.g.,
an improvement in wastewater treatment
efficiency) might be implemented solely as management actions and would be implemented with/without the adaptive management
learning opportunity. Other projects
(e.g., a monitoring program
to assess standards compliance)
are implemented solely to assess the
effectiveness of management actions. Still other projects
(e.g., implementation of a
particular agricultural BMP) might serve both purposes. For the
purposes of the value of information
analysis proposed here, the relevant
project cost is that which relates to the learning objective.
In estimating
the value of research, experimentation,
and monitoring, the decision maker must assess the utility for reduction in
TMDL forecast uncertainty. Some
decision makers may be
able to directly consider the merits
of uncertainty reduction, but many
probably will not. For a decision maker, reduced TMDL forecast uncertainty
is meaningful when translated into
social and economic consequences, but these may not be readily apparent. Thus, for this proposed value-of-information
scheme to work effectively, the
analyst should be prepared to discuss the expected
outcomes associated with reduced
uncertainty to stimulate thinking on
the part of the decision maker concerning the socioeconomic consequences of “good/bad”
decisions. Similar difficulties are likely to be encountered
when the decision maker estimates the weights on the attributes. Fortunately, there are ways to
present the cost/uncertainty trade-offs that may
result indirectly in the estimation
of these weights. In sum, these are difficult choices, and many decision makers lack experience with
these choices; however, that does not change
the fact that implicit in decisions to undertake/forego new information gathering is an assessment of these terms in this decision model.
Finally, there may be essentially an unlimited number of projects that might
be considered for learning; in
practice, it is feasible to rigorously assess only a handful. It seems reasonable
to assume that scientists and water
quality modelers will be able to
identify proposed management actions that have the greatest
uncertainty concerning impacts, they
should know the uncertainty in the
forecasted outcome (and can easily compute the value of compliance monitoring), and
they should know (or be able to assess) the weakest components of the model that might
be improved with experimentation. Thus, in practice, we are
likely to depend on the judgment of the scientific analysts to identify a relatively small number
of options for consideration in this
procedure.
The expected utility analysis described here can
help prioritize proposed research and monitoring
to reduce uncertainty in predicting the impact
of management options for water quality decision making, including but not limited
to TMDLs. The role of research and monitoring within a resource management setting is to reduce selected
uncertainties to an acceptable level
so that appropriate resource management
decisions can be made/improved. Scientists are too-often criticized for
hesitating to predict results of management
options, instead calling for further
study. The approach described here provides a framework for assessment,
planning, and research that may
enlighten the choice to fund research, to implement management
actions, or to do both.
No comments:
Post a Comment